Cascade learning has changed the automation game, attaining long-desired reliability with near 100% success rates in item picking.
Vision AI enabled the automation of many complex, monotonous and dangerous tasks, but the reliability of most vision AI powered robotic cells has long disappointed. Early iterations provided lackluster performance below a reasonable usability threshold, with excessive error rates. The introduction of supervised machine learning to vision AI improved performance, but most cells relying on this technology still make erroneous picks approximately six out of every 100 tries when handling tasks like parcel induction or order fulfillment. Even the world’s largest e-commerce marketplaces still fail more excessively when picking transparent poly bags and handling other highly complex tasks. While some early adopters learned to live with these shortcomings, others waited for more acceptable performance, suppressing industry adoption of vision AI for variable piece picking. Cascade learning has changed the game, attaining long-desired reliability with near 100% success rates in item picking. Automated Packaging System
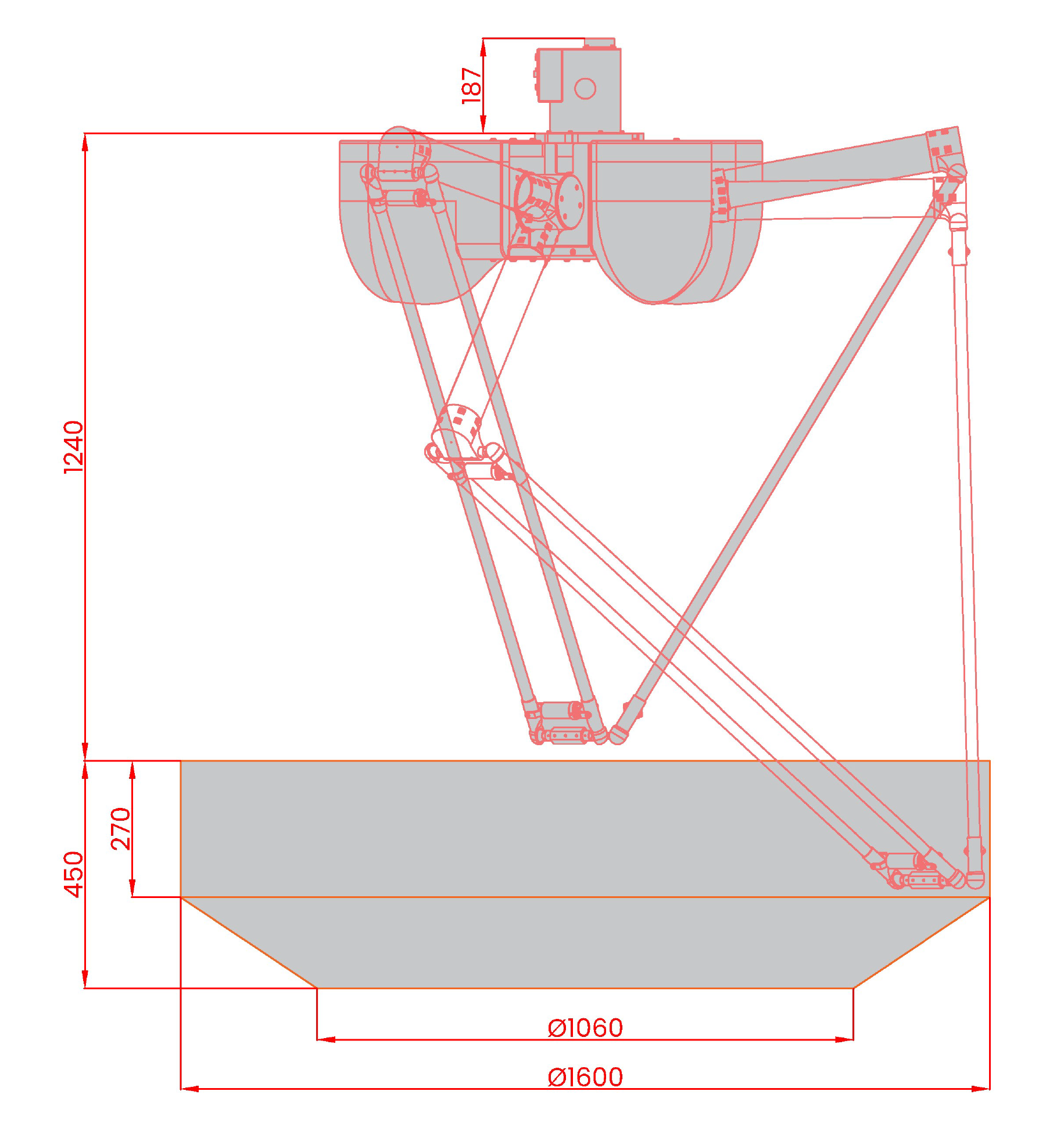
Cluttered environments and variance-filled tasks require robots that can see and process quickly and accurately; vision AI touts many well-established examples of complex tasks that can now be automated:
At the bleeding edge of this innovation, loose loads trailer and container unloading represents the next frontier. Loose loads offer supply chain and logistics pros a greener solution that can reduce carbon emissions since loose loads can fill trailers up to 96% versus palletized loads that can only fill trailers approximately 60%. However, older robotic cells often rely on traditional computer vision and top out at 70-80% success rates. Newer installations that integrated supervised machine learning still struggle with unacceptable error rates despite improvements. This leads to goals only being partially achieved. The goal, for example, might be for 10 robots to complete 10 jobs independently, but far more often, these 10 robots will require a few human supervisors to intervene and deal with miss-picked and double-picked items. In each case, automation has fallen short of its true potential.
Cascade learning teaches vision AI to process data from images according to a cascading set of considerations and takes supervised machine learning to new heights, erasing the error rates plaguing computer vision. Success rates in mature applications that use cascade learning can reach 99.99%, limiting robotic mistakes to one in every 10,000 picks. Performance improvements via cascade learning become vast and rapid as operators control what, how and when robots learn, including which variables to consider and in what order, removing the need for robots to unlearn incorrect behaviors. In an unsupervised situation, de-learning and re-learning poses a problem for most vision AI. Identifying the source(s) of faulty information from which incorrect behaviors were learned can present significant challenges; the information must be removed, and the robot retrained. The ability to direct how, what and when robots learn provides the invaluable control required to greatly simplify this process.
In advanced vision AI, cascade learning should be integrated throughout a three-step process that empowers a robot to receive critical information from an image and direct a robot to take the appropriate action with a gripper in milliseconds: Step 1: Identification After a camera captures an image, the vision AI uses segmentation, shape detection and material detection to identify the task at hand. Segmentation allows the robot to understand where one item ends and another begins, and shape detection clarifies how much space each item takes up on a three-dimensional plane, whether it’s a box or an envelope, etc. Material detection allows the robot to know what each item is made of, whether that’s cardboard, poly bag, a hard plastic container or something different. Step 2: Prioritization Cascade learning makes its most impactful contribution during prioritization. Cascading layers of consideration must be taught and incorporated into the underlying algorithms. During prioritization, the vision AI combines two-dimensional and three-dimensional data and uses sequencing to determine which items are on top of others. It also analyzes the surface of each item to locate the flattest, largest, sturdiest or otherwise most desirable surfaces with which to work. Among other considerations, it determines whether its gripper will have adequate room to move, where it must begin its task, etc. Processing these considerations in milliseconds keeps the line moving and bottlenecks at bay. Step 3: Robot communication With a sophisticated plan of attack ready to accomplish the task at hand, the vision AI then sends instructions to the robot. These include pick positions, the proper angle of approach, selecting the right force and gripper options out of a set of configurations, and more. All these instructions are communicated according to the specifications of each robot’s coded capabilities.
Many recent installations across Europe and North America showcase the reliability and flexibility of cascade learning as a foundational element of supervised machine learning in vision AI. These installations raise the bar and set new standards with greater than 99.9% success rates. Errors sometimes occur just once in 10,000 picks. DHL robotizes in its parcel sorting center AWL recently empowered DHL Parcel Benelux to pick and place parcels from randomly mixed pallets onto a sorting installation conveyor belt. The robot can lift up to 31.5 kg and process 800 parcels per hour. This unique solution handles a wide range of parcels at high speed. GRIPP can pick 200,000 parcels in a week Inther Group recently unveiled its Gantry Robotic Intelligent Piece Picker (GRIPP), a fully automatic compact picking technology that can pick up to 1,200 items per hour 24 hours a day, seven days a week. GRIPP “picks up products precisely and optimally regardless of their shape by means of the adaptive approach angle and highly intelligent, flexible and self-learning software.” Heavy bag depalletizing AVT deployed multiple depalletizing robots for two different end users that pick and place heavy bags from variable pallet stacks, automating one of the most difficult and hard to staff jobs in the supply chain and providing a critical competitive advantage to food manufacturers and other dry goods processors. See one in action in this video. Intelligent robot depalletizing Arvato Supply Chain Solutions’ new intelligent robot depalletizer, Stark, handles random mixed boxes with label recognition and singulation and has been installed both in the United States and Europe. Motoman manages parcel induction Yaskawa’s Motoman picks and places parcels onto conveyors for rapid handling of boxes, poly bags and padded envelopes of varying sizes to enhance parcel handling operations, deliver exceptional productivity gains and reduce operational costs.
Ken Fleming is the CEO of Fizyr, which offers advanced computer vision for robots, providing the smartest, fastest and most effective brain available to maximize robotic capabilities.
Check out our free e-newsletters to read more great articles..

Sorting Machines ©2023 Automation.com, a subsidiary of ISA